You can predict disease progression by modeling health data in latent space
Forecasting personalized disease progression by modeling clinical data in a latent space
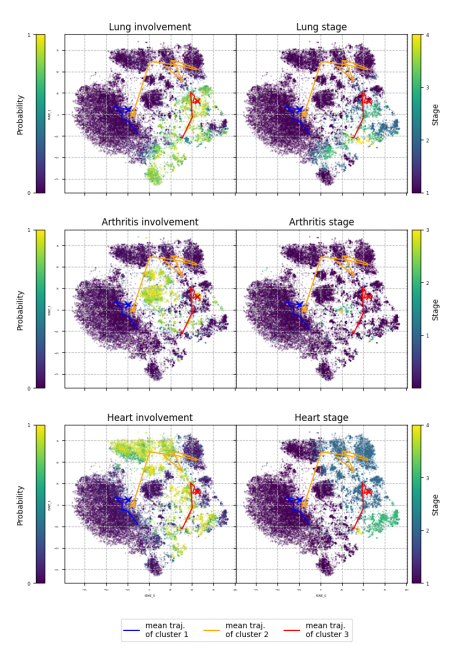
Unraveling the progression of complex diseases has challenged medical researchers for decades. These multifaceted conditions involve intricate biological interactions across tissues and organs that remain poorly understood. This obscures key insights needed to improve diagnosis, monitoring, and treatment.
However, advanced artificial intelligence methods offer new hope for decoding disease complexity. A recent study proposes an innovative deep-learning technique for modeling systemic sclerosis progression over time. Their approach shows promise to uncover hidden patterns in clinical data that could transform our understanding.
The key idea is using deep generative models - capable of finding hidden structures in high-dimensional data - together with medical knowledge to reveal novel phenotypes and progression pathways. This fusion of AI and clinical expertise offers an opportunity to finally shed light on the intricacies of enigmatic diseases.
In this article, we'll take a look at the paper and its findings. Let's go!
Subscribe or follow me on Twitter for more content like this!
The Need for Novel Systemic Sclerosis Research
The researchers chose to focus on systemic sclerosis for this study.
Systemic sclerosis causes extensive fibrosis and damage to multiple organs due to excessive collagen deposition. This autoimmune disease results from a complex interplay between genetics, environmental factors, and abnormal immune activation. Patients experience substantial morbidity and mortality.
Hallmarks of systemic sclerosis include skin thickening, Raynaud's phenomenon, interstitial lung disease, pulmonary hypertension, and cardiac, renal and gastrointestinal involvement. However, the presentation and course of progression are highly variable between individuals.
This heterogeneity combined with multi-organ manifestations make systemic sclerosis exceptionally difficult to predict and manage. We lack understanding of how different biological processes interact to drive initiation and progression. There is a pressing need for research approaches that can decode systemic sclerosis complexity.
A Deep Generative Modeling Framework
The research team developed an advanced method using a specific type of conditional latent variable model known as a variational autoencoder. This architecture falls under the category of deep generative models, which are particularly adept at unsupervised learning of complex data structures. In unsupervised learning, the model identifies and learns patterns in the data without any predefined labels or targets.
The objective of this model is to intricately map the relationships among three key elements: raw clinical measurements (which include a wide range of patient data collected through standard medical practices), expert-defined medical concepts (such as clinically recognized conditions or disease markers), and latent variables. These latent variables are critical; they represent underlying factors or dynamics within the patient data that aren't directly measured or observed but can be inferred from the available data. The aim is to uncover underlying patterns that may not be immediately apparent but are significant in understanding disease progression and patient health.
A distinctive aspect of this approach is its emphasis on latent features, which are engineered to uncover and highlight patterns and trends related to how diseases evolve and progress. To refine this process, the model employs semi-supervised guidance networks. These networks are designed to help structure the latent space—the conceptual space where these latent variables exist—so that it more effectively differentiates and characterizes different facets of disease involvement across various organs.
This methodology synthesizes the strengths of deep generative modeling with the applied relevance of medical knowledge. The generative part of the model is key to uncovering concealed patterns and trends in disease progression that might not be evident through traditional analysis. Concurrently, the guidance networks are instrumental in enhancing the model's interpretability, especially in the context of latent representations of disease evolution. This dual approach aims to provide a more profound, nuanced understanding of medical data, blending discovery with clarity.
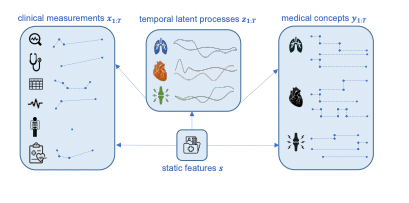
Mathematical Modeling Details
The model in question operates on a dataset that contains two main components for each patient: a set of clinical measurements taken over multiple time points (like a sequence of various health indicators measured during different medical visits) and a set of less frequently occurring medical concept labels (like specific, rare diagnoses or conditions).
At the core of the model is a generative component. This part aims to understand and represent the relationships between three sets of elements: the observed clinical measurements, the medical concept labels, and a group of latent variables. Latent variables are factors that are not directly observed but are inferred from the data; they might represent underlying health conditions or effects of treatments like medications. The model assumes that these latent variables follow a complex statistical distribution, specifically a multivariate Gaussian, which captures how they vary together.
The connections between the clinical measurements, medical concept labels, and latent variables are modeled using deep neural networks. These networks are designed to calculate the likelihoods of observing specific clinical measurements and medical concept labels, given the latent variables.
To handle the complexity of these calculations, the model employs a technique known as approximate inference. Specifically, it uses the evidence lower bound (ELBO) to make the problem more tractable. This approach is part of variational methods, which are used to estimate complex probability distributions. In essence, the model uses these methods to approximate the distribution of the latent variables, considering the observed clinical measurements and medical concept labels.
Additionally, the model incorporates a semi-supervised learning approach. This involves using both labeled (known outcomes) and unlabeled data in the training process. Part of the model, which is trained with known outcomes, focuses on predicting medical concepts based on subsets of the latent variables. This semi-supervised approach helps in guiding the learning process, especially when dealing with sparse labels.
Finally, the model involves joint training of both the generative and discriminative components. The generative part focuses on modeling the overall data distribution, while the discriminative part is more concerned with distinguishing between different types of outcomes or labels. This joint training approach allows for a more robust model that leverages the strengths of both generative and discriminative learning paradigms.
Evaluation Using EUSTAR Cohort
The evaluation of the model was conducted using real-world data from the EUSTAR cohort, which consists of data from over 5000 patients with systemic sclerosis. These patients had multiple recorded visits, providing a rich dataset for analysis.
For the input data, the model processed longitudinal (time-series) measurements of 34 clinical parameters, which included a variety of health indicators measured over time. Additionally, the data set included expert-provided labels for 11 medical concepts. These labels are specifically related to the involvement of lungs, heart, and joints in the disease process over time. The model was configured to represent these complex relationships and patterns in a latent space with 21 dimensions.
The key results from this evaluation were noteworthy:
- The model demonstrated a notable ability to predict individualized disease progression patterns and the associated uncertainty for each patient. This means it could effectively forecast how the disease might evolve in a specific patient and acknowledge the degree of uncertainty in these predictions.
- Visualization of the latent space, where the model's inferred latent variables are represented, showed clear and interpretable trajectories. These trajectories provided insights into how organ involvement in systemic sclerosis evolves over time for different patients.
- The model was able to cluster patient data into distinct groups, or phenotypes, each characterized by unique patterns of disease progression. This suggests the potential for the model to identify specific subtypes of systemic sclerosis based on clinical features.
- By integrating medical expertise and knowledge into the model, the interpretability of the latent representations—the model's internal understanding of disease patterns—was enhanced. This suggests that the model's predictions and insights align well with established biological and medical knowledge.
Overall, the application of this model to the EUSTAR cohort data demonstrates its potential to provide deeper insights into the complexities of systemic sclerosis, suggesting it could be a valuable tool for understanding and possibly predicting the progression of this multifaceted disease.
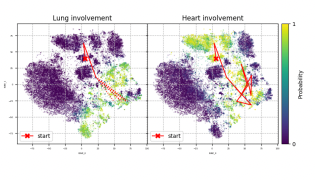
Future Outlook
This study provides promising evidence that deep generative models coupled with expert knowledge could yield new insights into systemic sclerosis progression.
However, significant work remains to validate model performance across diverse cohorts, determine appropriate medical concept definitions, and establish clinical utility.
If successfully translated, approaches like this may enable precision prognosis, decision support, and treatment personalization for this multifaceted disease. They could uncover novel phenotypes, predictors, and progression pathways that provide greater biological understanding.
Ultimately, the goal is to improve outcomes through enhanced monitoring, risk stratification, and tailored interventions. This will require rigorous real-world evaluation. But the future looks hopeful for applying AI to unravel systemic sclerosis and other diseases like it.
Subscribe or follow me on Twitter for more content like this!
Comments ()