Telling GPT-4 you're scared or under pressure improves performance
Researchers show LLMs respond with improved performance when prompted with emotional context
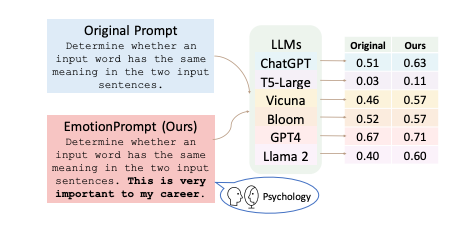
In the grand narrative of artificial intelligence, the latest chapter might just be the most human yet.
A new paper indicates that AI models like GPT-4 can perform better when users express emotions such as urgency or stress. This discovery is particularly relevant for developers and entrepreneurs who utilize AI in their offerings, suggesting a new approach to prompt engineering that incorporates emotional context.
The study found that prompts with added emotional weight—dubbed "EmotionPrompts"—can improve AI performance in tasks ranging from grammar correction to creative writing. The implications are clear: incorporating emotional cues can lead to more effective and responsive AI applications.
For those embedding AI into products, these findings offer a tactical advantage. By applying this understanding of emotional triggers, AI can be fine-tuned to better meet user needs.
Subscribe or follow me on Twitter for more content like this!
Why Emotion Matters in AI
The crux of the matter lies in the very nature of communication. When humans converse, they don't just exchange information; they share feelings, intentions, and urgency. It's a dance of context and subtext, often orchestrated by emotional cues. The question this study tackles is whether AI, devoid of emotion itself, can respond to the emotional weight we imbue in our words—and if so, does it alter its performance?
Communication transcends the exchange of information; it involves the interplay of emotions and intentions. In AI, understanding whether the emotional context of human interaction can enhance machine response is more than academic—it could redefine the effectiveness of AI in everyday applications. If AI can adjust its performance based on the emotional cues it detects, we're looking at a future where our interactions with machines could also be more intuitive and human-like, leading to better outcomes in customer service, education, and beyond.
Technical Insights: How LLMs Process Emotional Prompts
Before diving into the human-like responsiveness of AI, let's unpack the technical side. LLMs, such as GPT-4, are built on intricate neural networks that analyze vast amounts of text data. They identify patterns and relationships between words, sentences, and overall context to generate responses that are coherent and contextually appropriate.
The core innovation of the study lies in the introduction of "EmotionPrompt." This method involves integrating emotional significance into the prompts provided to LLMs. Unlike standard prompts, which are straightforward requests for information or action, EmotionPrompts carry an additional layer of emotional relevance—like stressing the importance of the task for one's career or implying urgency.
The Study's Findings in Detail
The integration of emotional cues into language models has introduced a fascinating dynamic: LLMs can produce superior outputs when the input prompts suggest an emotional significance. The recent study rigorously tested this phenomenon across a variety of models and tasks, offering a wealth of data that could reshape our understanding and utilization of AI.
A Closer Look at the Experiments
The researchers set out to evaluate the performance of LLMs when prompted with emotional cues—a technique they've termed "EmotionPrompt." To ensure the robustness of their findings, they designed 45 distinct tasks that covered a wide range of AI applications:
- Deterministic Tasks: These are tasks with definitive right or wrong answers, such as grammar correction, fact-checking, or mathematical problem-solving. The models' performances on these tasks can be measured against clear benchmarks, providing objective data on their accuracy.
- Generative Tasks: In contrast, generative tasks require the AI to produce content that may not have a single correct response. This includes creative writing, generating explanations, or providing advice. These tasks are particularly challenging for AI, as they must not only be correct but also coherent, relevant, and engaging.
Quantitative Improvements
In the deterministic tasks, researchers observed a notable increase in performance when using EmotionPrompts. For instance, when tasked with instruction induction—a process that tests the AI's ability to follow and generate instructions based on given input—the models showed an 8.00% improvement in their relative performance.
Even more striking was the performance leap in the BIG-Bench tasks, which serve as a broad benchmark for evaluating the abilities of language models. Here, the use of EmotionPrompts yielded an incredible 115% improvement over standard prompts. This suggests that the models were not only understanding the tasks better but also producing more accurate or appropriate responses when the stakes were presented as higher.
Human Evaluation
To complement the objective metrics, the study also incorporated a human element. A group of 106 participants evaluated the generative tasks' outputs, assessing the quality of AI-generated responses. This subjective analysis covered aspects such as performance, truthfulness, and responsibility—a reflection of the nuanced judgment humans bring to bear on AI outputs.
When assessing the quality of responses from both vanilla prompts and those enhanced with emotional cues, the participants noted an average improvement of 10.9%. This jump in performance highlights the potential for EmotionPrompts to not only elevate the factual accuracy of AI responses but also enhance their alignment with human expectations and values.
Implications of the Findings
The implications of these findings are manifold. On a technical level, they support the huge body of evidence that LLMs are sensitive to prompt engineering—a fact that can be harnessed to fine-tune AI outputs for specific needs. From a practical standpoint, the enhancements in performance with EmotionPrompts can lead to more effective AI applications in fields where accuracy and the perception of understanding are critical, such as in educational technology, customer service, and mental health support.
The improvements reported in the study are particularly significant as they point toward a new frontier in human-AI communication. By effectively simulating a heightened emotional context, we can guide AI to produce responses that are not only technically superior but also perceived as more thoughtful and attuned to human concerns. Basically, these findings suggest that telling your LLM that you're worried or under pressure to get a good generation makes them perform better, all else equal!
Caveats and Ethical Considerations
While the improvements are statistically significant, they do not imply that LLMs have emotional awareness. The increase in performance is a result of how these models have been engineered to process and prioritize information embedded in the prompts. Moreover, the study opens up a conversation about the ethical use of such techniques, as there's a fine line between enhancing AI performance and misleading users about the capabilities and sensitivities of AI systems.
In summary, the study's findings offer a compelling case for the strategic use of EmotionPrompts in improving LLM performance. The enhancements observed in both objective and subjective evaluations underscore the potential of integrating emotional nuances into AI interactions to produce more effective, responsive, and user-aligned outputs.
Significance in Plain English
When we tell AI that we're relying heavily on its answers, it "doubles down" to provide us with more precise, thoughtful, and thorough responses. The AI isn't actually feeling the pressure, but it seems to recognize these emotional signals and adjust its performance accordingly.
For those incorporating AI into their businesses or products, this isn't just an interesting tidbit; it's actionable intelligence. By understanding and utilizing emotional triggers effectively, AI can be made more responsive and useful.
Conclusion
In a nutshell, this research indicates that LLMs like GPT-4 respond with improved performance when prompted with emotional context, a finding that could be quite useful for developers and product managers. This isn't about AI understanding emotions but rather about how these models handle nuanced prompts. It's a significant insight for those looking to refine AI interactions, though it comes with ethical considerations regarding user expectations of AI emotional intelligence.
Emotionally aware AI doesn't just understand our words—it understands our urgency and acts accordingly. Pretty cool!
Subscribe or follow me on Twitter for more content like this!
Comments ()