Decoding Speech from Brain Waves - A Breakthrough in Brain-Computer Interfaces
Researchers from Meta have discovered how to turn brain waves into speech using noninvasive methods like EEG and MEG
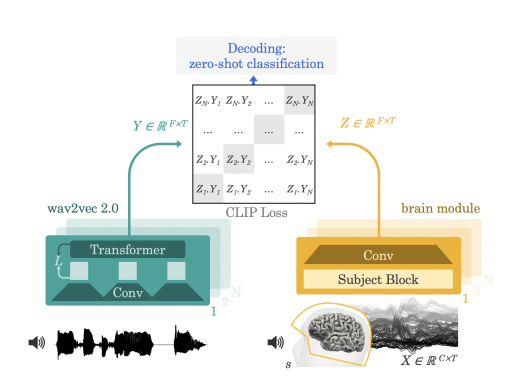
A recent paper published on arXiv presents an exciting new approach to decode speech directly from non-invasive brain recordings. This could pave the way for restoring communication abilities in patients who have lost the capacity to speak due to neurological conditions. The study provides hope that with continued research, non-invasive brain decoding could give a voice to the voiceless.
How did they figure out how to turn brain recordings into speech? Let's see.
Subscribe or follow me on Twitter for more content like this!
The Challenge of Speech Loss
Being unable to communicate can be devastating. Every year, thousands of people lose the ability to speak due to brain injuries, strokes, ALS, and other neurological conditions. Patients become trapped inside their own minds, unable to express their thoughts, feelings, needs, and desires. This profoundly diminishes their quality of life and takes away their autonomy and dignity.
Restoring speech is an extremely difficult challenge. Invasive brain-computer interfaces that implant electrodes in the brain can allow patients to type with their thoughts. But synthesizing natural speech from brain signals - without electrodes - has remained elusive.
A Novel Speech Decoding Approach
In this new study, researchers used a deep learning model to analyze non-invasive brain recordings as participants passively listened to speech. Electroencephalography (EEG) and magnetoencephalography (MEG) sensors captured the brain signals.
The model was trained to predict representations of the speech audio from the corresponding brain activity patterns. This allowed it to decode speech by matching new brain recordings to the most likely speech representation.
Three key innovations were involved:
- Using a contrastive loss function for training proved more effective than traditional supervised learning approaches. This loss encouraged the model to identify speech latents that were maximally aligned with the brain latents.
- Leveraging powerful pretrained speech representations from the wav2vec 2.0 model provided richer speech data than hand-engineered speech features used previously.
- A convolutional neural network tailored to each participant's brain data with a "subject layer" improved individualization.
The model was trained on public datasets comprising 15,000 hours of speech data from 169 participants. Also of note: testing on new unseen sentences demonstrated an impressive zero-shot decoding ability.
Significant Improvements in Accuracy
For 3-second segments of speech, the model could identify the matching segment from over 1,500 possibilities with:
- Up to 73% accuracy for MEG recordings
- Up to 19% accuracy for EEG recordings
This represents a dramatic improvement over previous attempts at speech decoding using non-invasive sensors. It also approaches the accuracy achieved in studies using invasive brain implants.
At the word level, the model achieved 44% top accuracy in identifying individual words from MEG signals. This ability to decode words directly from non-invasive recordings of neural activity is a major milestone, even at 44% effectiveness.
The Potential to Restore Natural Speech
This research provides hope that with sufficient progress, speech-decoding algorithms could one day help patients with neurological conditions communicate fluently.
Rather than surgically implanted electrodes, EEG and MEG sensors could potentially listen to the brain's intention to speak. Advanced AI could then synthesize the words and sentences on the fly to give a voice to the voiceless.
Hearing their own voice express unique novel thoughts and sentiments could help restore identity and autonomy to patients. It could really improve social interaction, emotional health, and quality of life.
Remaining Challenges
While extremely promising, many challenges remain before this technology is ready for medical application. The biggest one is that the current accuracy, while far beyond previous attempts, is still too low for natural conversations.
What's more, brain signals during active speech production may differ considerably from the passive listening scenario tested here. Further research on datasets recorded while participants speak or imagine speaking will be needed to ensure models are accurate.
Finally, EEG and MEG signals are susceptible to interference from muscle movements and other artifacts. Robust algorithms will be needed to isolate the speech-related neural signals.
A Milestone on an Important Frontier
This study represents a milestone at the intersection of neuroscience and artificial intelligence. Leveraging powerful deep learning approaches and large datasets, the researchers have pushed the boundaries of what is possible in decoding speech from non-invasive brain signals.
Their techniques provide a solid foundation for further advances. With rigorous research and responsible development, this technology may one day help restore natural communication abilities to patients suffering from neurological conditions and speech loss. This is an important milestone on the long road to giving a voice back to the voiceless.
Subscribe or follow me on Twitter for more content like this!
Comments ()